Visit UCR Return to Campus website - Take the COVID Screening Check survey
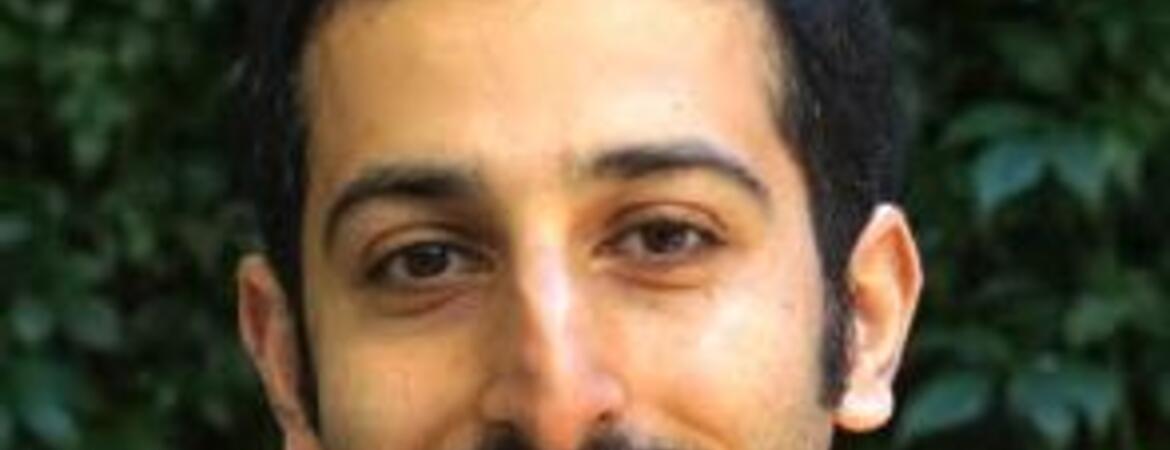
Title: Safety and resilience of large-scale networks via contraction theory
THURSDAY, MARCH, 3, 2022 11:00AM-11:50AM
Abstract: Recent decades have witnessed the rise of network systems that operate with unprecedented scale and efficiency. Examples include smart infrastructures, robotic networks, and artificial neural networks. However, providing safety and reliability guarantees for these networks is usually challenging due to their large size and their inherent nonlinearity. In this talk, we present elements of a non-Euclidean contraction theory to establish stability and robustness certificates for neural networks and dynamic flow networks. In the first part of the talk, we study the input-output robustness of implicit neural networks with respect to adversarial perturbations. Implicit neural networks are a recently-developed class of learning models that replace the layers in traditional neural networks with a fixed-point equation. Inspired by a dynamical system perspective to implicit networks, we study the solvability of their fixed-point equations via contraction theory and provide (i) tight conditions for their well-posedness, (ii) explicit bounds on their input-output Lipschitz constants, and (iii) optimization problems for their robust training. Numerical simulations show the improved robustness of our implicit neural networks compared to state-of-the-art architectures. In the second part of the talk, we study the resilience of dynamic flow networks with respect to transient uncertainties. By extending the classical contraction theory, we establish a dichotomy in the asymptotic behavior of dynamic flow networks. Using this dichotomy, we investigate the resilience of the input metering in transportation networks.