Visit UCR Return to Campus website - Take the COVID Screening Check survey
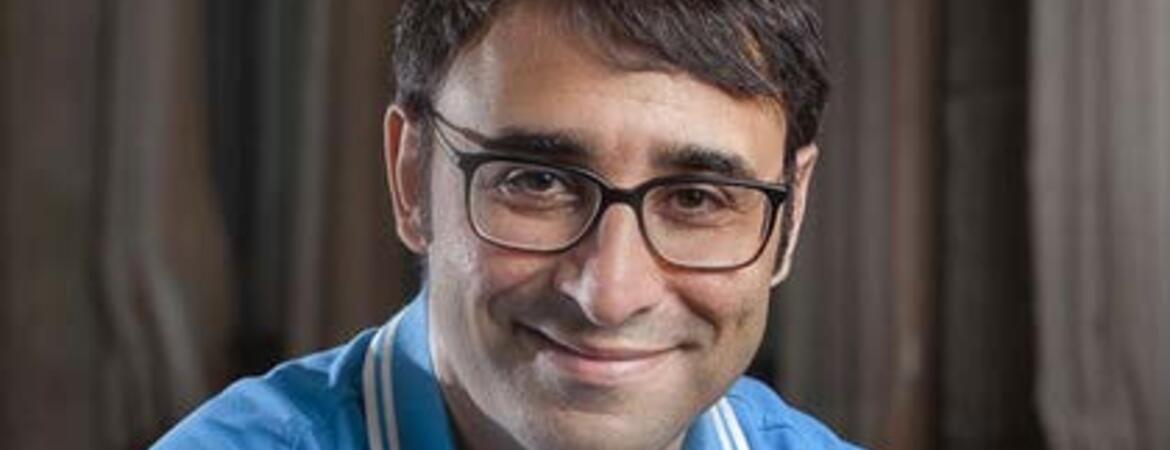
Title: Universal approximation power of deep neural networks through the lens of control theory
THURSDAY, APRIL, 28, 2022 11:00AM-11:50AM
Abstract: The talk is concerned with a key problem in the intersections of machine learning and autonomy: when is it possible to provide formal guarantees when deep learning is used within the control loop? We explain the universal approximation capabilities of deep residual neural networks using tools from control theory. In particular, we show that residual networks with a large class of activation functions have the power of universal approximation. The first contribution consists of relating the universal approximation problem to the controllability of an ensemble of control systems corresponding to a residual network, and to leverage classical Lie algebraic techniques to characterize controllability. The second contribution is to identify monotonicity as the bridge between controllability of finite ensembles and uniform approximability on compact sets. We describe how these results can play a key role in developing stability guarantees for neural networks in the loop.